이번 포스팅에서는 Pytorch를 사용하여 Modeling하는 내용을 작성할 것이다. 먼저 Modeling할 때 기반 클래스가 되는 nn.Module과 Conv, Linear, Sigmoid, Dropout등의 다양한 모듈을 살펴본 후, forward와 parameter에 대해서 다룰 예정이다.
1. 모델 클래스 생성
■ nn.Module
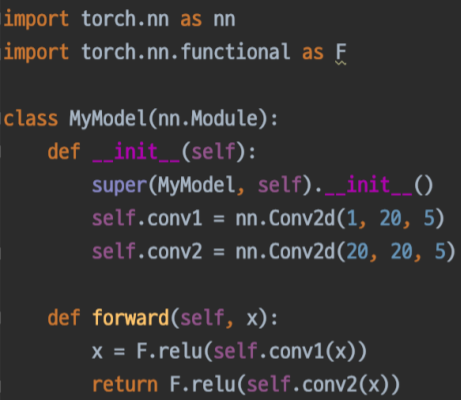
위 코드와 같이 Pytorch에서 Model 클래스를 생성할 때 nn.Module클래스를 상속하는데, nn.Module에는 Pytorch에서 사용하는 자료형인 Tensor클래스 및 forward, backward 등의 학습에 필요한 Function클래스와 같은 다양한 클래스와 메서드가 포함되어 있다.
■ modules
자신이 설계하는 Custom모델의 __init__메서드에서도 nn.Conv2d, nn.Linear 등 다양한 nn.모듈들을 사용할 수 있다.
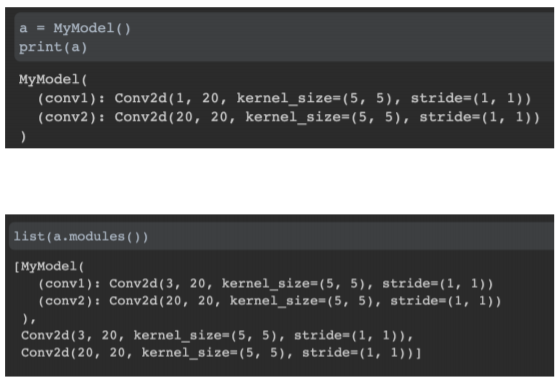
자신이 설계한 모델의 인스턴스를 print로 출력하면 모델의 구조가 나온다. 그 모델에 사용된 모듈을 확인할 때는 .modules() 함수를 이용할 수 있다.
■ forward
forward는 말 그대로 순전파 계산을 말하며, 자신이 설계한 모델의 인스턴스에 data를 넣어 호출하면 forward가 실행된다. forward를 실행시키는 부분은 __call__메서드에 있기 때문에 a.forward(x)로 명시하지 않고 a(x) 호출만으로 실행할 수 있다.
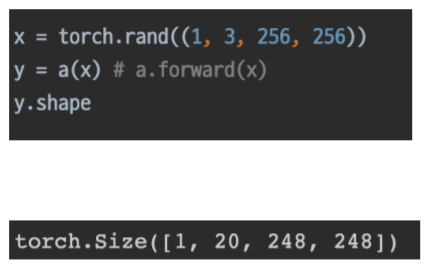
■ Parameter
Parameter는 __init__에서 정의한 Layer들의 weight, bias같은 파라미터를 저장하기 위한 클래스이며 data, grad, requires_grad 등의 변수를 가지고 있다. Parameter클래스가 존재하는 이유는 backpropagation, optimizer단계에서 '학습에 필요한' 변수를 그렇지 않은 일반 tensor로부터 구분함으로써 parameter만을 학습하기 위함이다.
.state_dict() 또는 .parameters() 함수를 통해서 모델에 존재하는 parameter들을 확인할 수 있다.


2. Transfer Learning
■ Pretrained Model
Pretrained Model이 학습한 데이터는 바로 ImageNet이다. ImageNet은 약 14milions개의 이미지와 20thousands 카테고리로 구성되어 있다.
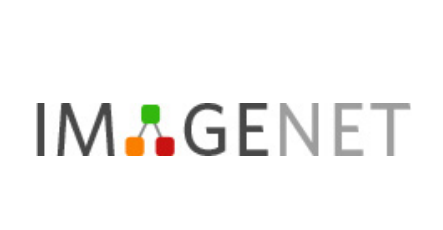
ImageNet 데이터셋이 등장한 후로 딥러닝은 급속도로 발전하기 시작했다. ImageNet Pretraining은 ImageNet데이터로 각 모델 및 classifier를 훈련하여 실생활에 존재하는 이미지를 1,000개의 class로 분류하는 것이다.


위 그림에 있는 수많은 모델들은 이 ImageNet으로 학습을 진행한 모델이다. 좋은 품질의 대용량 데이터로 미리 학습한 모델을 가져와 목적에 맞게 다듬어서 사용하면 훨씬 효율적이고 좋은 성능을 가질 수 있다. 현재 성능이 검증된 수많은 모델들이 있으며, 그 모델의 구조와 weight를 손쉽게 가져올 수 있다.
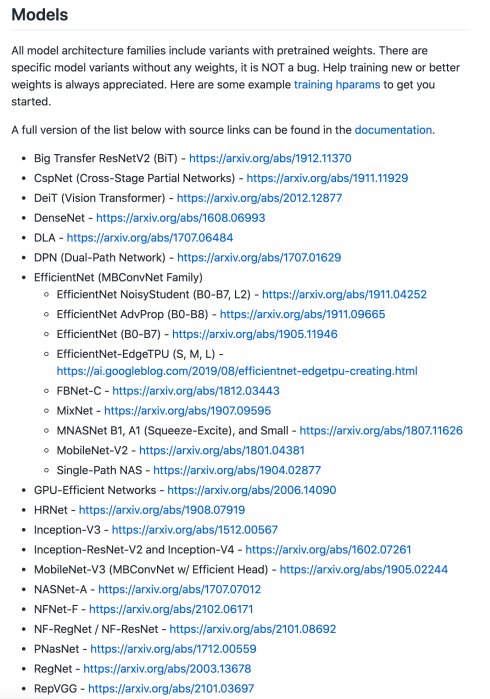
■ Transfer Learning
이미지 분류 문제의 경우, Transfer Learning할 때 데이터를 Input시키고 Backbone모델과 classifier로 훈련 및 분류를 하여 결과를 도출한다.

· 학습데이터가 충분한 경우
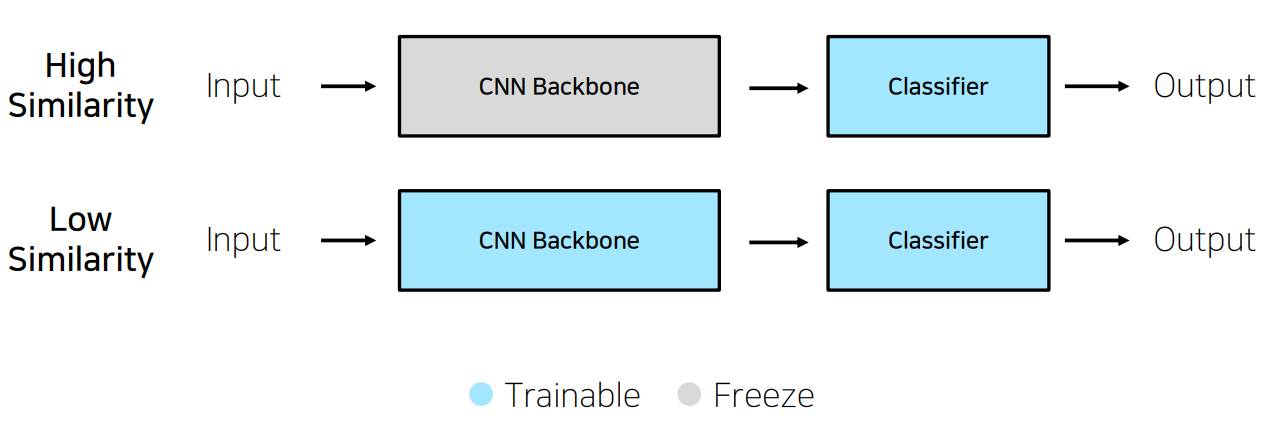
학습 데이터가 충분하고 pretrained model과 현재 해결하고자 하는 Problem Definition의 유사성이 높은 경우
→ Backbone의 parameter는 freeze시키고, classifier부분의 parameter만 해결하고자 하는 문제의 class에 맞도록 train한다.
학습 데이터가 충분하고 pretrained model과 현재 해결하고자 하는 Problem Definition의 유사성이 낮은 경우
→ Backbone의 구조만 사용하고 Backbone과 classifier의 parameter 둘 다 새로 train한다.
· 학습데이터가 충분하지 않은 경우
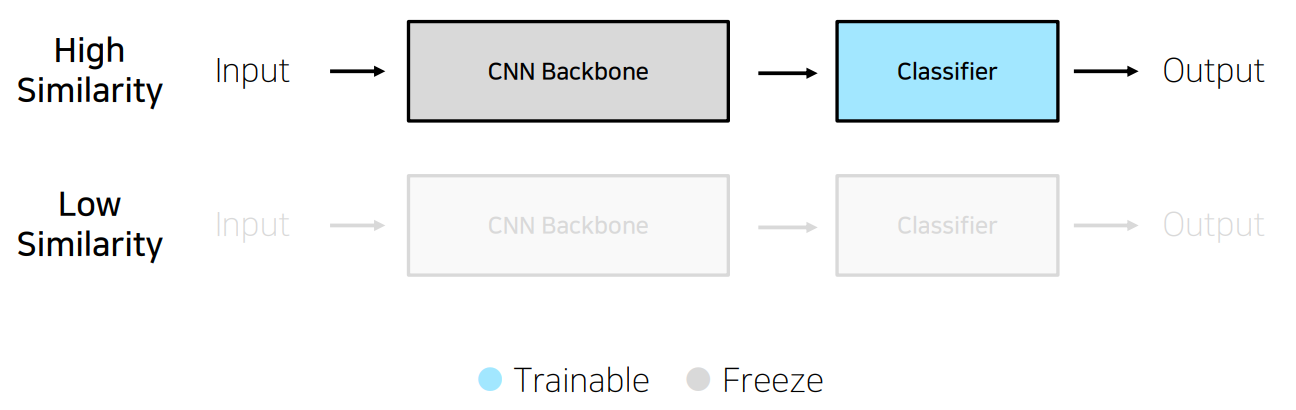
학습 데이터가 충분하지 않고 pretrained model과 현재 해결하고자 하는 Problem Definition의 유사성이 높은 경우
→ Backbone의 parameter는 freeze시키고, classifier부분의 parameter만 해결하고자 하는 문제의 class에 맞도록 train한다.
학습 데이터가 충분하지 않고 pretrained model과 현재 해결하고자 하는 Problem Definition의 유사성이 낮은 경우
→ Transfer Learning을 권장하지 않는다.
'AI > 딥러닝' 카테고리의 다른 글
[NLP] Seq2seq with Attention (0) | 2021.09.10 |
---|---|
[NLP] NLP Tasks , Bag of Words , Word Embedding , GloVe (0) | 2021.09.09 |
[이미지 분류] Data Processing (0) | 2021.08.24 |
[Pytorch] Dataset , DataLoader (0) | 2021.08.22 |
[Pytorch] Hyper-parameter Tuning , Pytorch Troubleshooting (0) | 2021.08.21 |
댓글